Learning behavioral manifolds for recognition and synthesis
We consider the problem of inferring the behavior of a target system given
cues in the form of a time-series taken from another co-evolving system. We
show how to approximate the target system's dynamical manifold with a
probabilistic model, then compute trajectories on this manifold that are
optimal w.r.t. the target system's dynamics and w.r.t. configural clues in
the cue signal. The resulting method integrates information over the entire
time-series to disambiguate many-to-many mappings between cue configurations
and target configurations, yet can run in linear time. We demonstrate with
systems that infer 3D nonrigid motion from image cues and, in the case of
facial motion, from audio.
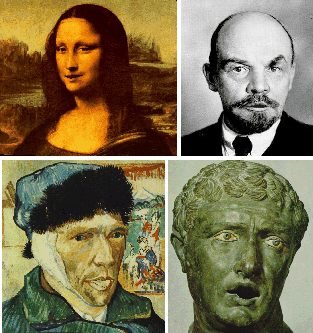